It is now well accepted that animals are sentient beings, with the commonly-farmed domestic species known to be capable of a complex variety of intellectual capacities, and of experiencing positive and negative emotions (Nawroth et al, 2019). Keepers of farm animals and consumers of livestock products are therefore invested in the idea that good welfare of farmed animals is desirable and an ethical responsibility (Weary and von Keyserlingk, 2017). Traditionally, commonly used frameworks in the UK such as the Five Freedoms have focused on the prevention of negative experiences. More recent frameworks for animal welfare assessment however have expanded this to include positive experiences and capacities such as choice and decision-making (Mellor, 2016). The Farm Animal Welfare Committee suggested in their 2009 review, Farm Animal Welfare in Great Britain: Past, Present and Future, that policy goals should be to ensure that all farm animals have a ‘life worth living’ and an increasing number should enjoy ‘a good life’.
Farm animal welfare is currently safeguarded by a number of mechanisms in the UK, not least by the compassion and empathy of those responsible for their animals' care, but also by legal statute and industry-lead farm assurance schemes and associated assessment protocols. Until relatively recently most welfare standards consisted of ‘input’ measures, which typically focus on an animal's access to essential resources (e.g. feed space, lying space), and on practices likely to be detrimental to welfare (e.g. disbudding without sufficient pain relief). In addition, there has also been adoption of ‘outcome’ measures in the last decade by various health and welfare assurance schemes, such as ‘AssureWel’ (a collaborative project between the University of Bristol, the RSPCA and the Soil Association (Van Dijk et al, 2018)). Outcome measures tend to incorporate animal-centric metrics associated with animal welfare, such as disease and mortality incidence, mobility and condition scores, and prevalence of post-mortem lesions. Both ‘input’ and ‘output’ measures are arguably necessary in any credible welfare scheme or assessment that attempts to estimate the welfare experiences of an animal or group of animals over the course of their life.
However, those critical of farming systems have argued that current welfare assessment mechanisms do not adequately address the positive emotional aspects of welfare; it is not enough to say that animals are productive, have access to basic resources, and are largely in good physical health, because such animals may still lead emotionally unrewarding, unhappy lives. In cattle production systems, this is often an argument mobilised in the debate surrounding access to grazing vs. continuously housed systems. There is a need, therefore, to develop outcome measures for assessing animals' emotional wellbeing that can feasibly be applied on farm.
Assessing animals' emotional health
To date, attempts to monitor animals' emotional state have focused on physiological measures considered to be associated with emotion (e.g. heart rate variability, cortisol in blood/hair/dung, body temperature), as well as behavioural measures considered to reflect positive or negative emotion (e.g. ear or tail position, play behaviour, avoidance/approach latency) (Reefmann et al, 2009). Physiological measurements are currently outside the reach of most formalised welfare schemes, although the increasing amount of technology used on farms and the likely reduction in system costs, may bring some of these within reach in the short to medium term. Behavioural measures may be more straightforward to assess and can also be quantified, in the sense that the frequency of a particular posture or behaviour can be counted, or an avoidance or flight zone can be scored (Murphy et al, 2014). Here too on-farm agri-tech offers future possibilities for measurement, by creating individual and herd time budgets for relevant movements, postures and behaviours, and picking up signs of emotional disturbance such as pain. However, monitoring behaviour to assess emotion can be challenging in that key indices might only occur infrequently (e.g. play), or may be difficult to interpret. Some behaviours are unequivocal in their emotional valence (e.g. mutual grooming), but the meaning of others for welfare can be more difficult to gauge, such as whether or not a certain number of feed-face displacements reflects undesirable aggression.
Qualitative behaviour assessment as a potential assessment tool
In this evolving field of animal welfare concepts and methods, an additional angle has been provided by a method called ‘qualitative behaviour assessment’ (QBA), which is based on observing the dynamic whole animal, rather than isolated physical aspects of its behaviour (Wemelsfelder et al, 2001; Wemelsfelder, 2007). The main idea underlying QBA is that an animal's demeanour, the way in which it moves around, conveys a dynamic expressive quality (a ‘body language’) reflecting its experience of its surroundings; movement can, for example, have a relaxed, curious, fearful, or dejected quality (Figure 1). Our ability to ‘read’ an animal's — or human being's — mood this way is a skill that holds a lot in common with good stockmanship, based as it is on attunement to an animal's or herd's character, and the ability to judge when the animal/herd's expressions deviate from the norm. Consider walking into a shed of cows, one of which has a left-displaced abomasum: there are few cattle veterinary surgeons who would not be able to pick out the affected animal simply from the way the animal holds itself or reacts to an unfamiliar person appearing (even before individual clinical signs are consciously noted). QBA does not merely ‘measure behaviour’, it is a form of integrative qualitative assessment of an animal's condition and demeanour in which appreciation of context plays a crucial role.
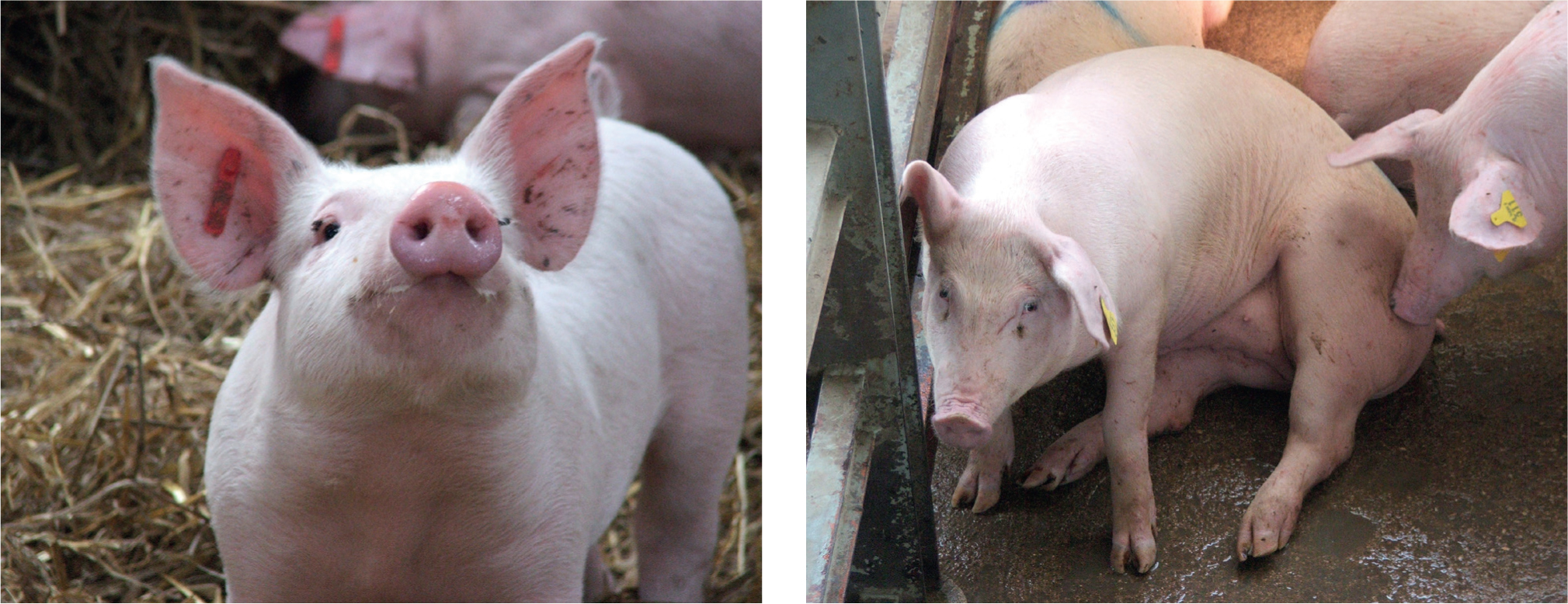
However such skills, despite their practical value, have long been regarded as ‘subjective’ human judgements of uncertain validity. One concern, for example, is that the less physically similar we are to a species, the harder it becomes to read its emotions (Würbel, 2009). Yet a growing body of QBA research by science teams across the world has found that assessments of emotional expressivity in a range of animal species correlate well with measurements of their physical health, physiology and behaviour (e.g. Stockman et al, 2011, 2013; Rutherford et al, 2012; Phythian et al, 2016; De Boyer des Roches et al, 2018). Stockman and colleagues (2013), for example, recorded video footage of cattle that were naïve to road transport, and again after habituation to transport 9 days later, and found that the cows' mood scores significantly correlated with physiological stress measures, such as core body temperature, heart rate, plasma glucose and neutrophil:lymphocyte ratio. There is thus growing acceptance that qualitative assessments can be as much about the biological animal as quantitative ones — as long as appropriate training and methodology are provided. QBA provides a ‘whole animal’ type of information that is complementary to knowledge gained from more specific health and welfare measures; ideally the different types of assessment should strengthen each other as part of larger farm assurance schemes (Main et al, 2014).
QBA involves an assessor observing for some time (10–20 minutes) how individuals or groups of animals engage with each other and their environment, and then scoring the individual's or group's expressive demeanour on about 20 descriptors. Scoring relies on unstructured visual analogue scales (VAS), using either preagreed terms selected by an assessor group, or terms adopted from previous initiatives. An emotion is typically graded from complete absence — for example ‘not at all aggressive/happy/interested’ — to ‘the most aggressive/happy/interested’ that can be imagined (Figure 2).
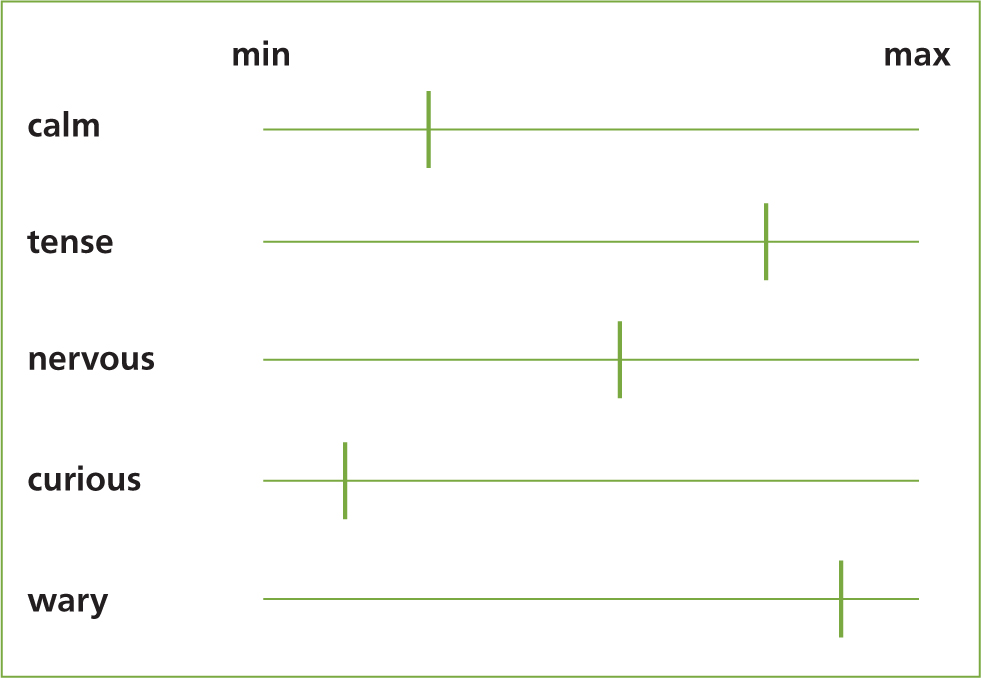
Scores are collated by measuring the distance along the line between the minimum point and the assessor's mark, and are then analysed using principal component analysis (PCA). PCA is a commonly used multi-variate statistical technique that looks for clustering between variables (i.e. terms) to determine underlying dimensions explaining most of the variation between measured items (i.e. farms/animals). Whichever terms correlate most strongly with the emergent dimensions determine their meaning. In on-farm settings the primary emerging dimensions tend to range from positive to negative mood (e.g. content–nervous) and from low to high energy expression (e.g. calm–excited), together forming four quadrants of expressivity widely recognised as capturing emotional range in animals and humans (Mendl et al, 2010). PCA ascribes scores to individual animals or herds on each of the primary dimensions, thus locating them in one of the four quadrants and characterising their emotional state relative to the other herds/animals in the sample. Because QBA is not based on absolute numerical values, and PCA detects patterns of variation within a given sample, it is important to acknowledge the relative nature of such characterisations; a particular herd may qualify as relatively anxious/nervous in a group of high-welfare farms, but as relatively content in a poorer group (Fleming et al, 2016). However, that something as complex as emotional wellbeing cannot be measured in absolute terms does not mean such characterisations are empty of meaning. The larger the sample of farms, and the greater the experience of an assessor, the more representative and well-honed an assessment of a herd's emotional health is likely to be.
Strong and vulnerable aspects of qualitative behaviour assessment
All methods have strong and weaker sides, pros and cons, and it is important to acknowledge these and work with them to optimise a method's efficacy. A primary USP of QBA is its directness in addressing animals as sentient beings, attending to the ‘whole animal's’ emotional expressivity rather than relying on post-hoc integration of component behaviours such as ear position or flight distance. Because it is so direct it is relatively time efficient to do, and can usually be completed within 20 minutes depending on the number of animals or groups being observed. Thus it can be incorporated into assurance scheme assessments quite easily.
Its directness also means QBA is likely to resonate with consumers, assessing animal feelings in a common sense, easy to grasp language. Although QBA's analytical techniques probably do not interest consumers, the fact that emotional health is of concern to the industry and considered worth measuring is likely reassuring. For farmers productivity tends to be a dominant concern (Hubbard and Scott, 2011), however most farmers take great pride in their herd, and QBA could potentially provide a constructive gateway to help facilitate discussion around management changes that could benefit both a farmer's animals and business (e.g. provision of a loafing area, cow brushes, lameness prevention programmes). QBA could, furthermore, be used as a tool for training new staff in stockmanship skills (Ellingsen et al, 2014).
However the integrated nature of QBA assessments also brings vulnerabilities, most notably its open-ended, uncategorised measurement scale, the so-called VAS. Research has shown that assessors can in principle use such scales very effectively to assess emotional expression (Rutherford et al, 2012; Fleming et al, 2016); however people are known to vary in the way they calibrate these scales, and so in situations where different assessors contribute data to a farm assurance scheme, the challenge is to separate animal-generated from human-generated variation (Muri et al, 2017).
Various forms of bias (e.g. whether a farm is known to be organic or not) can potentially affect a person's scores (Tuyttens et al, 2014), and to minimise the effect of such externalities it is essential that assessors understand how to focus their assessment on the animals themselves, and are skilled judges of the many nuances of animals' expressivity. An earlier study, investigating the effect of visual background on QBA assessments, showed that when pigs were ‘green screened’ onto both enriched and barren environments, trained observers were able to ‘see through’ this background effect and not let it unduly affect their assessments of the pigs (Wemelsfelder et al, 2009). However, in addition to forms of personal bias, people may simply have different styles of calibrating the use of VAS. Some people dislike giving low scores, others never give high scores — such different scoring habits can lead to arbitrary differences between people in how they score an animal or herd. Research indicates, however, that such discrepancies can be overcome through focused efforts to align assessors' scoring styles in group training sessions (Grosso et al, 2016). Such training sessions should include discussion of the meaning of descriptors, and discussion and practice of the use of VAS, and should be held with some regularity to ensure consistency. Overall, with sufficient training and experience, assessors should be able to adjust any existing biases and learn to align and optimise the way they apply QBA to herds on farm.
Realisation of the potential of QBA currently requires investment in data information technology in order to optimise QBA's benefits and minimise its risks (e.g. through data normalisation). Farm assurance managers are not likely to have the time or interest to engage with complex statistical techniques such as PCA, and so, similarly to other health and welfare metrics, QBA analysis will need to be automated if it is to be practically useful; however, this should not include the actual observation and scoring of animals' expressions — QBA's aim is to encourage users to connect with animals as sentient beings and sensitise themselves to animals' emotional needs, and automating all of QBA would defeat this purpose. But recently a start has been made with applying mobile technology to QBA data collection and analysis, thanks to an Animal Welfare Seeding Award granted to Scotland's Rural College (SRUC) by Newcastle University on behalf of the Biotechnology and Biological Sciences Research Council (BBSRC) and the Animal Welfare Research Network (AWRN). Once this technology is in place, QBA can be tested and put to work as part of government, non-governmental organization (NGO), and business animal welfare certification schemes.
Conclusion
There is a need to extend our on-farm assessment of animal welfare beyond basic physical health, and include measures of emotional wellbeing as part of an animal outcome-centric approach. Provided assessors receive appropriate and regular training, QBA could, in conjunction with other welfare metrics, facilitate an economically efficient, meaningful and practical approach to animal welfare assessment, and readily be incorporated into producer group schemes and farmer discussion groups.
KEY POINTS
- Acknowledging farm animals as sentient beings requires development of indicators for their emotional wellbeing.
- Qualitative behaviour assessment (QBA) is potentially one such indicator, describing and quantifying the emotionally expressive qualities of how animals engage with their surroundings.
- Such ‘whole animal’ insight can complement information provided by more specific physical health and welfare measures as part of farm assurance schemes.
- QBA relies on ‘animal reading’ skills that hold much in common with good stockmanship; regular practice and training are essential for its reliable use.
- To realise QBA's potential for farm assurance, a start was recently made with applying mobile technology to its data collection and analysis procedures.