Lameness is an abnormal gait typically considered to be an expression of foot or limb pain. A meta-analysis found that the reported prevalence of lameness in UK dairy cows is approximately 30% (Afonso et al, 2020), meaning that lameness is a leading welfare concern in the dairy industry, although it should be remembered that beef cattle are also affected (Tunstall et al, 2019).
In addition to the negative welfare effects of lameness, the economic impacts can be considerable. In 2009, Willshire and Bell estimated that the ‘average’ lameness case cost individual dairy farms just over £320 and the collective cost to the UK dairy industry was estimated to exceed £120 000 000 per year (Willshire and Bell, 2009), but it is likely that these costs have increased since this work was published. A tool to calculate the cost of lameness on individual farms has been made available by the Agriculture and Horticulture Development Board (AHDB, 2023a) to help veterinary surgeons and farmers understand the economic impact that lameness is having on their farms. Entering example figures provides an illustration of the current economic effect of lameness: if average UK figures regarding herd size and productivity (AHDB, 2022; 2023b) are entered with a lameness prevalence of 10%, lameness is calculated to cost the example farm almost £11 000 per annum, primarily as a result of reduced productivity (Figure 1).
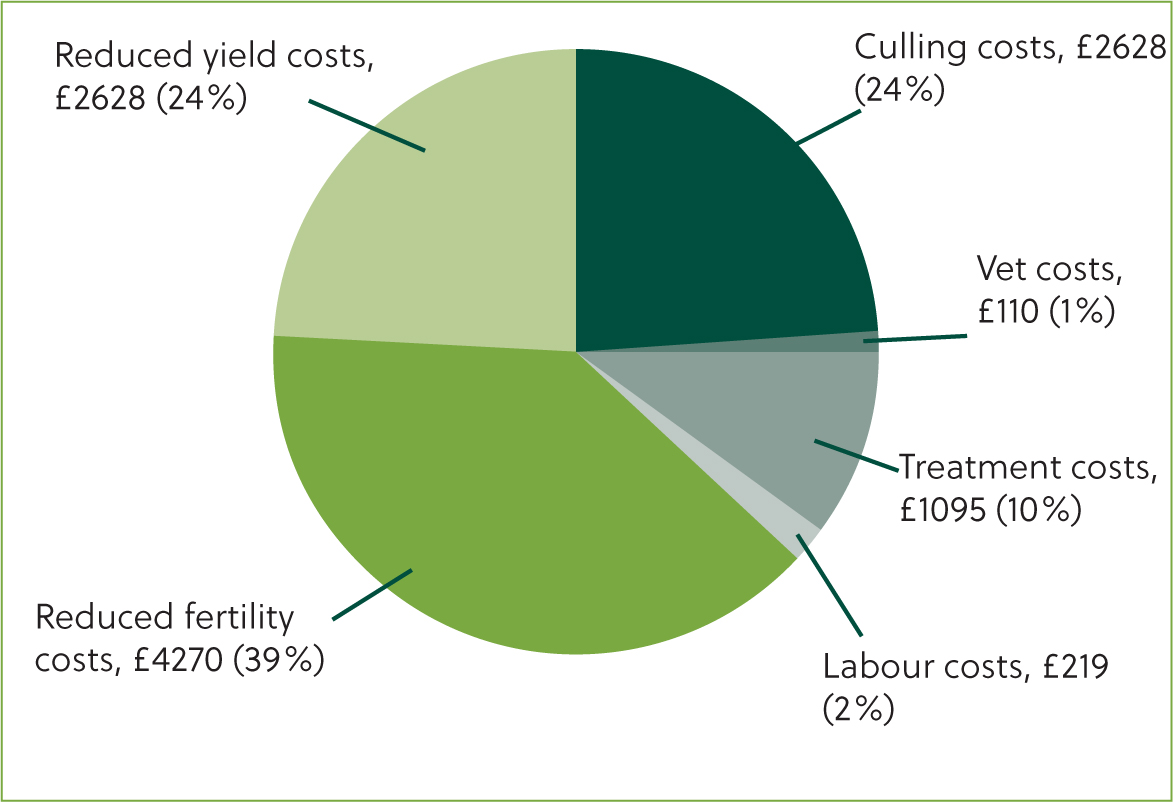
Visual observations are commonly used on farms for detection of lameness and typically involve assessment of gait for abnormalities indicative of limb pain, such as uneven weightbearing, back arching or head bobbing (Whay, 2002). However, while this method is inexpensive and simple to perform, it is time consuming and can be subjective. Furthermore, environmental factors such as floor type and condition can influence the gait of cows, affecting results (Van Nuffel et al, 2015a). For example, Chapinal et al (2011) recorded cows over several days walking on either rubber flooring or concrete and found that, when walking on rubber flooring, gait scores (scored using a 1 to 5 scale where 1 = perfect gait and 5 = severely lame) were lower and walking speed was increased compared to walking on concrete. To improve the performance of visual observations for lameness detection, it is recommended that a recognised scoring system is used (eg the AHDB mobility score; AHDB, 2023c). Several scoring systems are described (Whay, 2002; Van Nuffel et al, 2015a), and aim to reduce the subjectivity of visual observations by assigning a numerical grade to defined gait patterns — increasing number is typically associated with increasing severity of lameness. In the UK, the four-point AHDB mobility score (AHDB, 2023c) has been adopted as the industry standard for the past 15 years (Afonso et al, 2020). More recently, the Register of Mobility Scorers (https://roms.org.uk/) has been established in the UK. This organisation aims to promote good mobility scoring practices as well as attempting to standardise mobility scoring as much as possible through the use of trained, accredited personnel who undergo initial training followed by annual reaccreditation. Implementation of regular mobility scoring by trained personnel such as those on the Register of Mobility Scorers register is recommended to both monitor lameness prevalence and also aid prompt detection of lame cows.
Although expert consensus advocates regular scoring of lameness, this is often not done on farms; for example, Griffiths et al (2018) found that only 22% of farmers reported mobility scoring more frequently than quarterly and 35% of farmers did not mobility score at all. A study investigating farmers' attitudes to lameness management found that farmers reported not seeing a benefit of regular mobility scoring over ad hoc lameness detection and farmers who did feel regular mobility scoring would be beneficial cited practical difficulties as barriers to implementation (Horseman et al, 2014); findings that may explain the discrepancy between expert recommendations and uptake on farms. While severe lameness can be detected using ad hoc mobility scoring, it is more difficult to detect mild or moderate lameness in this manner. Furthermore, the ad hoc nature of this approach inevitably means that lameness assessment is not done at regular intervals; therefore, cows may be lame for some time before they are detected and treated — in particular more mildly affected cows (Horseman et al, 2014). Delays in detection and treatment of lameness lead to compromised cow welfare and can also adversely affect response to treatment (Thomas et al, 2016). The concept of ‘Early Detection, Prompt Effective Treatment’ (EDPET) has been developed with the aim of reducing delays in detection and treatment of lameness, thus interrupting the spiral of chronicity that can develop when lameness is not addressed promptly. This concept is now one of the four key pillars of the AHDB Healthy Feet Programme (AHDB, 2018), a framework intended to aid trained personnel to help farmers reduce the prevalence of lameness in their herds. Detailed discussion of EDPET is outwith the scope of this article but readers are referred to a review in this journal for more detail (Pedersen and Wilson, 2021).
The importance of early detection of lameness, together with the low uptake and subjectivity of regular visual observations (ie mobility scoring) has meant that there has been increasing interest in using technology to automate lameness detection on dairy farms. An additional benefit of automated lameness detection devices is their potential for routine monitoring of mobility of all cows (as opposed to merely identifying lame cows). There is the potential for this to be performed more frequently than is possible with visual observations, generating valuable data that can indicate trends in herd mobility over time. This article provides an overview of automated lameness detection with a focus on direct methods.
Automated detection of lameness
Several automated methods of lameness detection are available for use in cattle, typically using one (or a combination) of three methodologies (Table 1).
Table 1. Methodologies used in automated lameness detection systems
Methodology | Description | Example devices | |
---|---|---|---|
Direct methods | Kinematic gait analysis | Assessment of positional changes of body parts (eg feet) over time | High speed video technologyImage processingAccelerometers, pedometers |
Kinetic gait analysis | Assessment of forces applied | Ground reaction systemsPressure sensitive walkways | |
Indirect methods | Assessment of proxy measures of lameness related to pain, inflammation or disease | Thermographic imagingCow behaviourProduction parameters |
Machine learning is an approach that programmes algorithms to analyse and learn from data with the purposes of applying this knowledge to a designated task (such as lameness detection). How frequently the algorithm prediction is correct (termed classification accuracy and expressed as a percentage) is a commonly reported metric used to evaluate algorithm performance. Machine learning is often employed for automated detection of lameness; for example, Taneja et al (2020) applied machine learning techniques to accelerometer-generated data, finding that lame cows could be detected up to 4 days before they were detected by farmers (using visual observations) with an accuracy of over 80%. Similarly, Jiang et al (2022) applied machine learning techniques to video footage of back posture, identifying lame cows with an accuracy over 96%, and Warner et al (2020) successfully identified herds at high risk of lameness by applying machine learning to data obtained from farm records. As these studies demonstrate, machine learning techniques can be applied to data generated by both direct and indirect methods of automated lameness detection (Table 1), potentially offering a flexible and powerful tool for data analysis on farm in the future.
Pedometers and accelerometers
Pedometers are animal-worn devices containing a switch that is activated when the wearer moves, producing a signal indicative of the number of steps taken (Rutten et al, 2013). Accelerometers are also worn by the animal, but these devices contain a piezoelectric sensor which generates a signal proportional to the change in velocity (ie acceleration) experienced (Brown et al, 2013); generated signals are processed by the device software to produce an output that reflects animal movement. Modern accelerometers contain three piezoelectric sensors aligned to allow for capture of movement in three dimensions (Brown et al, 2013) and are also capable of detecting when the device is horizontally or vertically orientated, providing information regarding whether the animal is standing (vertical orientation) or lying (horizontal orientation). Accelerometer data are collected multiple times per second and collated to generate a continuous output; commercially available accelerometers used in the dairy industry typically employ a sampling rate of up to 40 samples per second (40 Hz). Accelerometers differ from pedometers in that they measure acceleration, whereas pedometers measure the number of steps taken; however, many commercially available accelerometers generate an output reporting both data, and the terms ‘pedometer’ and ‘accelerometer’ are often used interchangeably. Accelerometers and pedometers are already widely used in the dairy industry for oestrus detection (Stevenson and Britt, 2017) and studies suggest that animal-worn devices such as accelerometers are farmers’ preferred choice for automated lameness detection (Van De Gucht et al, 2017). Accordingly, these types of devices may have most potential for automatic lameness detection in a commercial setting.
Pedometers
Pedometers were first identified as potential aids to oestrus detection over 40 years ago (Kiddy, 1977) and are now well established in the dairy industry for this purpose (Stevenson and Britt, 2017), but the potential for pedometers to detect lameness is a more recent area of study and few data are available. Mazrier et al (2006) compared daily step count for individual cows with their average step count over the previous 10 days, finding that 46% of cows seen to have a reduction in pedometer activity subsequently developed clinical signs of lameness 7–10 days later. The remaining 54% of cows identified as having reduced pedometer activity were classified as false positive results (Mazrier et al, 2006), suggesting that poor specificity might limit the value of pedometers for the prediction of lameness. Changes in pedometer activity have been found to precede other conditions of dairy cows, such as mastitis and metabolic disorders (Edwards and Tozer, 2004; Kester et al, 2015) and are not specific to lameness; although, none of the remaining 54% of cows studied by Mazrier et al (2006) developed other diseases and the change in pedometer activity of these cows was unexplained. A greater than 5% reduction in pedometer activity was the threshold used by Mazrier et al (2006) to predict lameness, but 92% of cows that developed lameness had a reduction in pedometer activity of greater than 15%, leading the authors to suggest that a pedometer activity change of 15% may be a more suitable threshold for automated prediction of lameness (Mazrier et al, 2006). While the results reported by Mazrier et al (2006) suggest pedometers may have the potential to predict lameness, the detection of currently lame cows was not studied. Nevertheless, identification of cows that are of increased likelihood of developing lameness in the subsequent 7–10 days may enable farmers to direct time towards visual observation of these selected cows and allow early detection of lameness if it occurs.
Accelerometers
While accelerometers are more recent technology than pedometers, they have been rapidly accepted in the dairy industry for oestrus detection (Stevenson and Britt, 2017). Accelerometers are more widely used devices in studies of automated lameness detection than pedometers and were reviewed in detail by O'Leary et al (2020).
Accelerometer technology has been reported to have the potential to detect lameness with an accuracy of 100% (i.e. every lame cow was correctly identified and there were no false negatives or false positives) (Alsaaod et al, 2017); however, it should be considered that while this study highlighted the potential for accelerometer technology to aid lameness detection, cows wore two accelerometers (one on each hindlimb) and the accelerometers used were high frequency (400 Hz), a methodology not reflective of how accelerometers are currently used on commercial dairy farms. Low frequency accelerometers are more widely studied (Alsaaod et al, 2019) and have been reported to have potential for detecting lameness in cattle when worn on a single limb or on a neck collar, albeit with a lower accuracy than multiple high frequency accelerometers as described by Alsaaod et al (2017). For example, Alsaaod et al (2012) detected lameness with an accuracy of 76% using a leg-worn accelerometer. Similarly, Thorup et al (2015) found that a single leg-worn accelerometer could differentiate between lame and non-lame cows, as well as identify cows of different grades of lameness, but did not report accuracy of detection. A large degree of variation in activity between cows was reported in both studies (Alsaaod et al, 2012; Thorup et al, 2015), with Thorup et al (2015) also reporting a wide variation of activity between farms. As such, it is possible that comparison of a cow's activity data with her own ‘baseline’ (ie non-lame) data is likely to be more valuable than comparison to the herd average or a specified threshold to detect lameness in individual animals.
Video technology
Visual detection of lameness using video technology aims to replicate direct visual observations of animal movement. Flower et al (2005) first described the use of motion markers attached to the cow to analyse body movement and make comparisons between lame and non-lame cows. Cows were filmed walking in single file along a narrow walkway against a black background and the footage of healthy cows was compared to that of cows with sole lesions and sole ulcers (other causes of lameness were not studied). Cows with sole ulcers were found to have shorter strides of lower height than healthy cows, as well as reduced speed of walking, suggesting that this type of technology has the potential to reliably detect cows with sole ulcers (Flower et al, 2005). More recently, Van Hertem et al (2014) applied machine learning to analyse video footage obtained in a similar manner to Flower et al (2005), finding that while the system performed well when a binary lame/non-lame classification was used, performance was reduced if a 5-point lameness score classification was used (Van Hertem et al, 2014).
Studies using video technology to detect lameness have also investigated back posture as a possible lameness indicator, with promising results. For example, Viazzi et al (2013) found that machine learning was able to detect lameness using back posture with a high degree of accuracy. Interestingly — similar to studies of accelerometer-generated data (Alsaaod et al, 2012; Thorup et al, 2015) — large between-cow variation was observed and the system performed much better when an individual cow threshold was used to detect lameness compared to a herd-level threshold (91% accuracy vs. 76%) (Viazzi et al, 2013).
A video system marketed for lameness detection has become commercially available (Herdvision, Agsenze, UK) (https://herd.vision). This system comprises a camera positioned above the cow accommodation that detects lameness as well as changes in body condition score, transmitting data to the farmer's phone or tablet device. However, to the author's knowledge this device is yet to be externally validated.
Ground reaction systems and pressure walkways
Ground reaction systems
When lame, cows place less weight on the affected limb and more weight on the contralateral limb (Rushen et al, 2007). Ground reaction systems contain load cells that measure the forces applied to the ground as the cow walks, or individual limb weight distribution as the cow stands (Van Nuffel et al, 2015b), employing the principle of asymmetric weightbearing to detect lameness (i.e. lameness is detected if there is high variability in weightbearing or weight distribution between contralateral limbs) (Dunthorn et al, 2015). These devices commonly comprise a platform of fixed plates the cow either walks across or stands on, but recently a novel device worn by the cow has been described (Fischer et al, 2022).
Ground reaction systems can measure walking forces applied in one-dimension (1D) or three dimensions (3D). One-dimensional systems measure vertical pressure distribution only, whereas 3D systems measure pressure applied in three directions (vertical, latero-medial and cranio-caudal) (Van Nuffel et al, 2015b) and reportedly have superior lameness detection capabilities. However, data are only available from small studies (Alsaaod et al, 2019) and further work is merited; although data that are available are promising. For example, Dunthorn et al (2015) found a 3D system could detect lameness with both a sensitivity and specificity of over 90%, compared to 52% sensitivity and 85% specificity using a 1D system. A ground reaction system is commercially available (StepMetrix, Bou-Matic, USA) (Van Nuffel et al, 2015b; Stygar et al, 2021) but studies consistently report low sensitivity for detecting lame cows (Bicalho et al, 2007; Liu et al, 2011), possibly relating to alterations in how cows with painful limbs transfer loads when walking/standing (Van Nuffel et al, 2015b).
Devices developed to detect lame cows through individual limb weight distribution when standing also show promise (Van Nuffel et al, 2015b). Pastell et al (2006) found that lame cows could be reliably detected using this type of device but did not report sensitivity or specificity. A follow up study by the same authors expanded upon this preliminary work, reporting a high sensitivity (100%) but limited specificity (57.5%) (Pastell and Kujala, 2007). Nevertheless, these devices are reportedly easier to use (Alsaaod et al, 2019), and potentially do not require as much space as ground reaction systems that assess walking cows (Van Nuffel et al, 2015b), especially if they are incorporated into an automatic milking system; therefore, weight distribution systems may have greater potential for adoption on farms in the future than systems that assess walking cows.
Pressure walkway
A pressure walkway that combines kinetic and kinematic analyses to measure stride-related data (such as symmetry, length and step width) as well as forces applied has been developed (‘Gaitwise’) (Maertens et al, 2011), but is not yet commercially available in the UK. Maertens et al (2011) reported that the Gaitwise system was capable of detecting lameness with a sensitivity ranging from 76–90% and a specificity ranging from 86–100% depending on how severely lame the cow was (the system performed better at detecting more severely lame cows). These findings were supported by another study that found the Gaitwise system showed promise for detecting early lameness, but the authors did note that further study is needed to determine whether this system performs better overall than visual observations (Van Nuffel et al, 2013).
Alternative devices
A single study has reported the successful use of radar technology to detect lameness in cattle (Busin et al, 2019). Radars emit electromagnetic waves which, when obstructed (e.g. by a cow), are reflected back to a receiver that transforms the received signals into a meaningful output. When radar technology is used to detect animal or human movement, smaller bodily movements that occur during motion are also detected (Tahmoush, 2015). The signals generated by these smaller movements are called micro-doppler signatures and can be used to identify individual parts of the body (e.g. the legs). In the study reported by Busin et al (2019), cattle walked past a radar as they left the milking parlour and machine learning was used to identify differences in the micro-doppler signature of lame and non-lame cows. The system identified lame cows with a sensitivity and specificity of 85% and 81% respectively, although the system performed better when used to detect lameness in sheep (96% and 94% sensitivity and specificity respectively) (Busin et al, 2019). The authors suggest that this system has the potential for commercial use on farm because of its simplicity and expected cost-effectiveness; however, to date, this is the only study that describes this methodology for lameness detection and further research is warranted.
Application of automated lameness detection on farms
Development of commercial automated lameness detection systems is ongoing and has been widely reviewed (Alsaaod et al, 2019; Dutton-Regester et al, 2020; O'Leary et al, 2020). To be commercially viable, systems need to be acceptable to farmers, practical to implement and use, and economically feasible (Borchers and Bewley, 2015). Devices also need to be optimised for sensitivity and specificity of lameness detection, but studies do not always report these data making it difficult to assess their value for routine use. A study indicated that farmer preference was for animal-worn devices such as accelerometers and, as perhaps might be expected, farmers who already use these devices for oestrus detection were more accepting of their use for lameness detection (Van De Gucht et al, 2017). To date, one commercially available accelerometer in the UK is marketed for lameness detection which is combined with other features such as oestrus detection and lying times (‘Cow-Alert’; https://www.peacocktechnology.com/cowalert). Devices such as ground pressure reaction systems and video technology require more space than accelerometers (for example, a passage for the cows to walk through), which may limit their adoption on farms where space is typically at a premium. However, technological advances may lead to the development of smaller devices (such as the cow-worn pressure system described by Fischer et al (2022)) in the future that may promote wider adoption of ground pressure reaction systems.
Limitations of automatic lameness detection
It is important to recognise that — as failure of detection is only a partial contributor to the negative economic and welfare effects of lameness — on some farms, automated detection of lameness may not achieve significant changes in lameness prevalence (O'Leary et al, 2020). It is well documented that farmers commonly underestimate lameness prevalence in their herds (e.g. Leach et al, 2010); therefore farmers may not fully embrace automated lameness detection if they perceive there is limited benefit to be gained. Moreover, early detection of lameness must be followed up with prompt, effective treatment to successfully reduce the prevalence of lameness; however, delays in treatment are commonly reported and farmers may consider mild to moderate lameness to be non-urgent (Horseman et al, 2014), further delaying implementation of treatment. It also needs to be considered that high levels of farmer uncertainty and inadequate training of foot trimming have been reported (Tunstall et al, 2019; Pedersen et al, 2022), potentially resulting in ineffective or inappropriate treatment being provided to those lame cows that are detected. Therefore, to be effective, implementation of automated lameness detection systems needs to consider not just accuracy of detection but also other factors such as farm facilities, cost, and farmer training, as well as farmer motivation to reduce lameness prevalence through an integrated approach that effectively combines appropriate treatment with detection.
Conclusions
Lameness is a leading welfare concern in the dairy industry, with an estimated prevalence of 30%. While visual detection of lameness and regular (visual) mobility scoring are low-cost and straightforward to perform, they can be labour intensive, subject to error and difficult to implement on farm. Several automated detection of lameness systems are described, and all have the potential to assist farmers with lameness detection on farms; however, accelerometer technology may have the greatest potential for farmer acceptance as accelerometers are already widely used in the dairy industry for other purposes, such as oestrus detection. Although it is possible that the detection of lameness might be routinely automated in the future, detection needs to be followed up with prompt effective treatment of lame cows if effective reduction of lameness is to be achieved. As such, implementation of automated detection of lameness will need to be part of a wider approach to lameness on farm that includes introduction of appropriate treatment protocols to ensure that, when detected, lame cows are treated promptly and effectively.
KEY POINTS
- Lameness is common on dairy farms, with an estimated prevalence of approximately 30%.
- Visual observations are currently the most common method used to detect lameness, but can be labour intensive and subjective.
- Regular mobility scoring is recommended to detect lameness early but can be difficult to implement on farm.
- Automated detection of lameness has the potential to aid in lameness detection on farm.
- Several different methods of automated detection lameness are described, but not all are commercially available yet, or have been externally validated.