The use of artificial intelligence (AI) algorithms has become normalised in our everyday lives, from filtering our spam emails (Guzella and Caminhas, 2009) and suggesting which films to watch on Netflix (Koren, 2009; Töscher et al, 2009) to the advent of semi-autonomous vehicles (Ingle and Phute, 2016). The possibility to translate AI technology into production animal veterinary practice is extremely exciting and has the potential to transform how we operate as veterinary surgeons. The role of the farm animal veterinarian has evolved greatly over the last few decades, with a shift from routine procedures (such as disbudding) to a more preventative management role through the use of big data in cattle practice (Hudson et al, 2018). The list of critical farm animal veterinary equipment has shifted from having a disbudding iron in the car, to needing a laptop for herd health data analysis, and the farm vet of the future will no doubt further evolve to require additional tools and skills that leverage advances in AI for the improvement of animal health, welfare and productivity.
What is AI?
There is often a degree of confusion as to what ‘AI’ is; particularly with the ‘intelligence’ component, with it often being presumed that AI algorithms are truly intelligent, and able to ‘think’ as humans do. We are not yet at the stage of truly sentient algorithms, but they are able to mimic human outputs extremely accurately, such as the advent of ChatGPT. AI algorithms are often seen as a ‘black box’ where (contrary to conventional modelling techniques) the exact parameters utilised are not clear, but they largely learn in the same way that a veterinary student might do; learning patterns in data to make accurate decisions (Obermeyer and Emanuel, 2016), which we can subsequently test on novel data to measure the performance of the algorithms. There have been a range of medical applications of AI algorithms reported in scientific literature, including the accurate classification of skin cancer (Esteva et al, 2017), retinal disease (De Fauw et al, 2018), and haematological interpretation (Gunčar et al, 2018).
There is a range of publications exploring AI approaches to veterinary diagnostic imaging (Hennessey et al, 2022). While there is great potential for AI to accurately classify disease in a medical setting, these will only be effective for patients from a collaborative approach between data scientists and practitioners (Lynch and Liston, 2018), and although there are a large number of research projects showing the benefits of AI in the clinical management of patients, translation of this into clinical practice is far more seldom (Clifton et al, 2015). In livestock a commonly used term is precision livestock farming, which aims to provide real-time monitoring and management systems for farmers by combining different sensor technologies, data analytics and automation (Berckmans, 2017).
What is already available?
There are a range of monitoring systems already in practice on UK farms, including the prediction of high somatic cell counts (Ebrahimie et al, 2018) and the onset of calving (Fenlon et al, 2017). Sensor technology is being analysed to make decisions on anything from fertility to identifying disease through changes in rumination (Steensels et al, 2016). The use of automated feeding stations for animals represents an exciting opportunity for the early identification of disease; for example, the changes in feeding behaviour of calves being related to disease in calves (Borderas et al, 2009). Sensors could also be used to detect indicators of positive welfare states, such as play behaviour in calves (Carslake et al, 2020). In addition, there is also the potential for predictive models, including using somatic cell count to predict the the likelihood of recovery from a disease (Henderson et al, 2016). It is also possible to diagnose disease at a herd level such as with bovine viral diarrhoea (Machado et al, 2015) and the mastitis origin of a herd (Hyde et al, 2020).
There is great potential for autonomous AI algorithms in reducing labour demands for veterinarians and farmers, with one example being robotic milking units (Rodenburg, 2017). While there are always concerns with the effects of labour replacement on job security, far from replacing the job of the farmer, these technologies have the potential to liberate the modern proactive farmer from the more mundane physical tasks of dairy farming and moving them to a position of being able to act in a managerial role – a far better direction of labour. Automatically identifying disease and segregating animals for treatment has the potential to reduce manual labour, and potentially reduce animal handling stress in the same fashion.
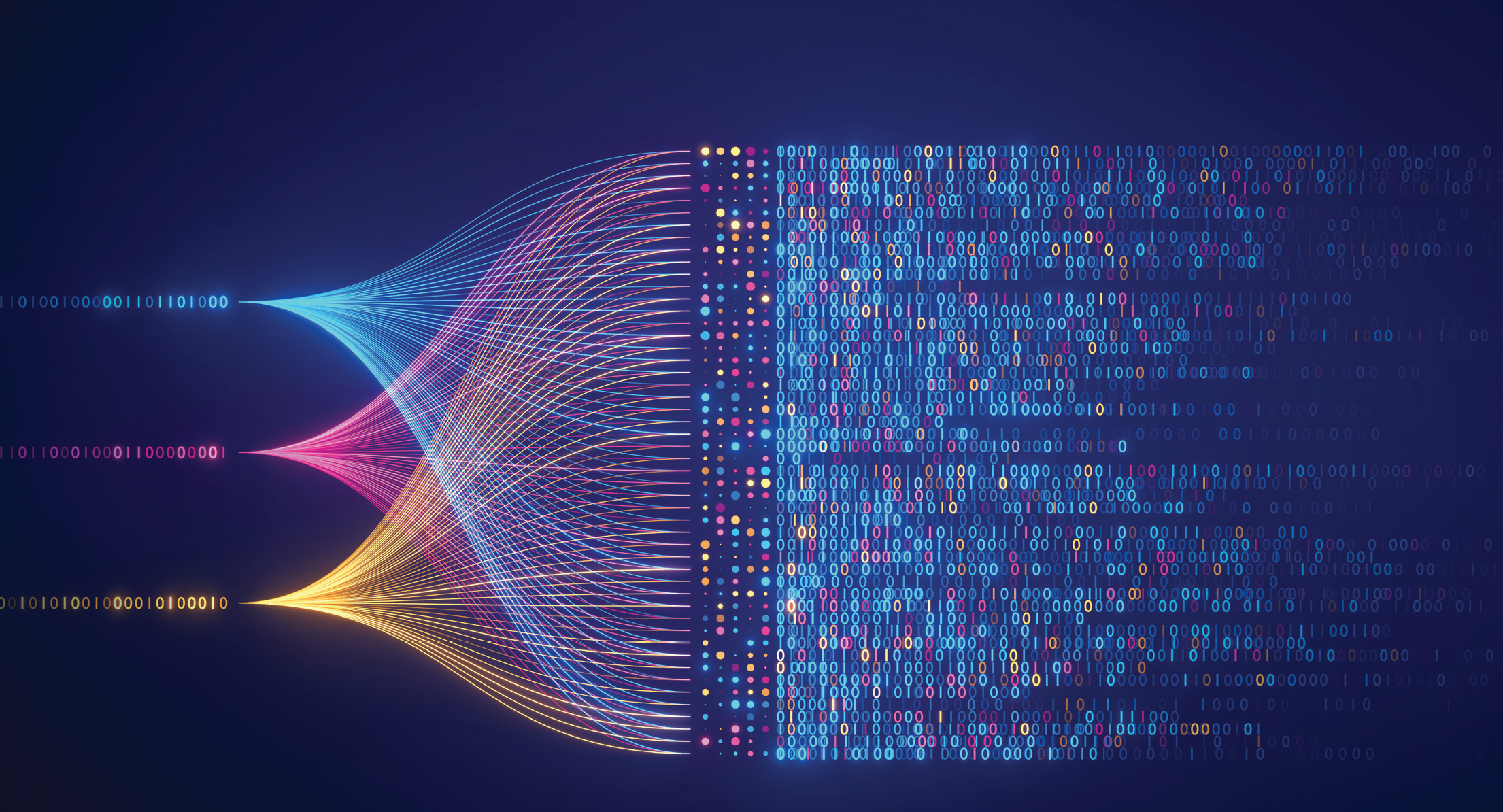
While sensors are commonplace, they can be limited in the information transmitted (Riaboff et al, 2022), often being based on accelerometer or XYZ coordinates. The advent of accurate computer vision algorithms means that detailed health and welfare behaviour can be monitored for groups of animals (Borges Oliveira et al, 2021) with a single camera, rather than requiring a single sensor per animal. Using cameras and AI to automatically monitor how livestock are behaving could provide vets and farmers with objective insights into livestock management, health and welfare. Such monitoring could pave the way for new veterinary consultancy services and performance monitoring. Computer vision can also help identify different diseases, such as the accurate identification of cattle lameness compared with human observers (Anagnostopoulos et al, 2023). There is a range of data skills that the production animal veterinary surgeon can learn to upskill in the age of AI (Hyde et al, 2021).
There are a number of challenges in developing AI algorithms, including the sheer volume of data from a myriad of sources; if we can pull all these together AI would have a better chance of learning patterns, but the fragmented nature of data including cattle movement records (and births/deaths), herd management software, milk recording organisation data, milking parlour/robot data, activity monitors, not to mention paper records, make this challenging (Hudson et al, 2018). Only by streamlining the currently disparate sources of data in effective pipelines will we unlock the true potential of AI algorithms.
Risks of AI
There are concerns about loss of jobs, but it should be anticipated that AI for veterinary surgeons will provide an incredible tool to improve their veterinary ability, shifting some of the more mundane elements of the job into more engaging tasks deciding what practical management changes to make depending on the data. There is certainly a risk of bias in AI, which has been seen in the human field with relation to race, gender and social status (Parikh et al, 2019). The risk of bias is a real possibility in production animal medicine, and AI trained on certain breeds, scenarios or in certain environments may not provide information that can be generalised to other animals or environments. There is a question as to whether a veterinary surgeon relying on AI for diagnosis would be responsible for a misdiagnosis, and there are a range of ethical questions around the responsible use of AI in veterinary medicine. There is certainly a need for regulatory oversight.
Trust in AI can be challenging, and in the autonomous driving literature it has been shown that even if driverless cars were accurate there are a multitude of reasons why people might still distrust their efficacy (Kaur and Rampersad, 2018). It is very hard to assess whether an AI algorithm is ‘good’ enough to trust as a vet would be trusted; an accuracy of 95% in classifying disease might sound excellent, but if the prevalence of disease in the dataset was 5% then the algorithm could completely miss all disease cases (0% sensitivity) and still end up with a 95% ‘accuracy’. It will be important for companies providing AI tools for vets and farmers to provide multiple performance metrics (such as sensitivity, specificity and balanced accuracy) to allow veterinary surgeons to make informed decisions. It is also important to avoid the ‘black box’ nature of some algorithms by providing the confidence interval of an algorithm to practitioners; a ‘negative’ diagnosis of anthrax by a (well-calibrated) algorithm with a confidence of 51% might be treated entirely differently to an equally ‘negative’ diagnosis of anthrax, but with a confidence of 99.999%.
The future of AI in production animal veterinary practice
There is no doubt that there have been, and will continue to be, incredible developments in the field of AI for animal health. The use of AI algorithms to diagnose/prevent disease in livestock will continue to advance, and the role of the veterinary surgeon will need to evolve to keep pace with these advances in technology. A further shift away from providing physical services to one of data management and interpretation is certainly possible. As more data and AI driven insights become available, it is likely that other stakeholders, such as welfare assurance schemes and product buyers, will want access to help verify and improve production standards. Vets will have a vital role to play in ensuring the veracity of these data and that insights are used to make tangible improvements to health and welfare. Indeed, while the accurate performance of AI algorithms to provide useful data is exciting, it is only useful (and often only safe) if it is interpreted and actioned upon by someone with a detailed holistic understanding of production animal health and welfare, and veterinary surgeons are well placed to fill this role.
Technological advances in the field of AI will happen whether veterinary surgeons are involved or not, and veterinary practitioners must decide whether they wish to be in the driver's seat, making the most of the potential of AI in revolutionising animal health and welfare, or simply as a passenger.