Many species of animal live in social groups, where complex relationships and structures form (Wey et al, 2008; Sueur et al, 2011). These social structures are an important attribute of an animal population (Whitehead, 2009), and the association patterns that occur will influence behavioural characteristics within social systems (Myers, 1983). Benefits of familiarity are gained from an individual's prior knowledge of a conspecific's behaviour, and in many social species it has been found that association patterns are non-random (Sueur et al, 2011), which suggests that animals generally prefer to associate with certain individuals.
The aim of this review is to summarise research based on how a tool called social network analysis (SNA) can be used to study the social behaviour patterns of dairy cattle. Applications based on the findings of such research are also discussed to facilitate enhanced management of dairy cattle, which can lead to increased welfare and productivity.
What is social network analysis?
SNA is being utilised with increasing prevalence to untangle patterns of social behaviour. Originally, this approach was used to aid the understanding of human relationships, but it has recently been adopted by animal behaviourists to observe association patterns in animals (James et al, 2009). This technique has been employed to understand the social networks of free-living species to reveal who is connected to whom within a study population, and shows an individual's position in their social group (Krause et al, 2007; Meise et al, 2019), and it has also been used to assess association patterns and social interactions in captive species (George et al, 2013; Rose and Croft, 2017; Lewton and Rose, 2020). It is thought that the structure of social networks within a population can be linked to environmental characteristics and the experience and personality of individuals (Croft et al, 2016).
The results of SNA are typically depicted within network diagrams called sociograms (Figure 1). These diagrams consist of nodes (individuals) and edges (associations), which show how individuals within groups are connected, and centrality measures are incorporated into the analysis to show the position and importance of certain individuals within a population (Table 1). Social patterns based on demographics, such as age of individuals, can also be applied to the networks.
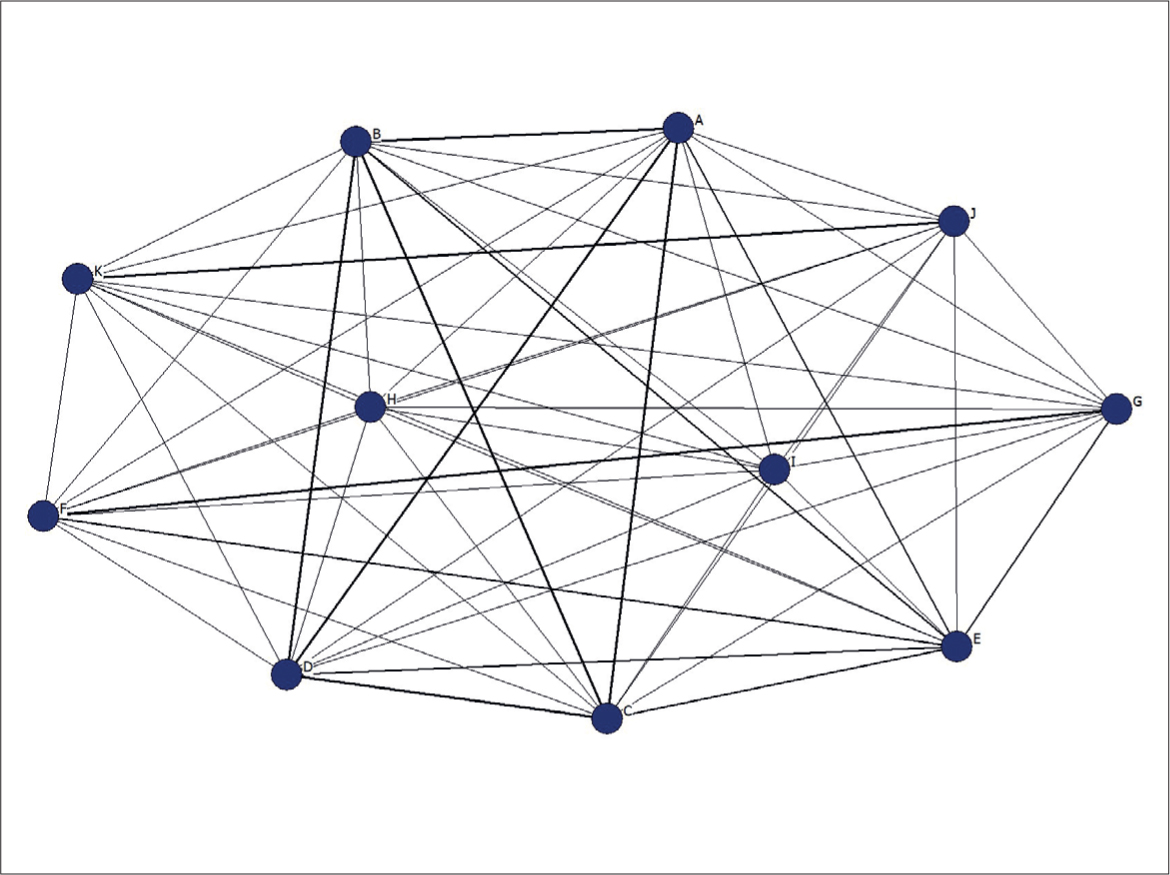
Table 1. Definitions of key SNA terms and centrality measures
Key SNA terms | Definition |
---|---|
Node | Refers to an individual in the network |
Edge | Represents an association or interaction between two individuals in the form of a line that connects two nodes |
Spring embedding | A method that is often used to show well-connected nodes in the centre of the network, and less well-connected individuals on the periphery |
Association strength | This refers to the strength of association between two nodes. It is the number of times two individuals associate during a specified time period |
Centrality measures | Definition |
Degree centrality | This refers to how many direct connections an individual has to other members of the population. Nodes with high degree values are usually well-connected and central to a network |
Closeness centrality | This is the sum of shortest paths that connect a node to all other individuals in the network. A node that is closely connected to others can interact without having to go through intermediaries |
Betweenness centrality | This is the number of shortest paths between any pair of nodes that pass through a focal node. Individuals with high betweenness may link different groups together |
Adapted from: Croft et al (2008); Whitehead (2008); Makagon et al (2012)
Although SNA has predominately been used to assess the social behaviour of wildlife and zoo animals, it can also be utilised on farm to understand social associations and interactions in livestock, such as pigs (Büttner et al, 2020), and it is an increasingly popular method for assessing the social behaviour of dairy cattle (Foris et al, 2019; de Freslon et al, 2019; Rocha et al, 2020).
Why is studying the social behaviour of dairy cattle important?
Cattle are hierarchical, and these hierarchies are subject to change depending on the addition or removal of individuals from a herd. Therefore, it is important to study the impacts of changes in social dynamics. For example, aggression is common during the flux associated with altering herd dynamics, so enabling established hierarchies to form is desirable and can lead to positive social behaviours, such as social grooming, which can reduce stress levels (Boyland et al, 2016). High stress levels can be an indicator of poor welfare, and understanding how cows interact can help identify individuals that may be instigating negative behaviours that may lead to acute or chronic stress in the herd. However, social behaviour can also be a key indicator of good welfare. For example, synchronised lying is a sign of an appropriate social environment (Fregonesi and Leaver, 2001), and this can be identified by monitoring the behaviour of individuals within herds.
A better understanding of social behaviour and social structure in dairy cattle can also instigate an improved provision of housing and enrichment for social groups. Social enrichment can easily be incorporated on farm to stimulate appropriate interactions (for review see Bolt and George, 2019), and the suitability of enrichment and housing design can be assessed by monitoring social behaviour. Dairy cow housing typically consists of feeding areas, lying areas, and space for activities such as loafing or grooming (Figure 2), and additional outside space is often provided (Figure 3), which allows for behaviours such as bulling (Figure 4). There are likely to be preferred areas within cattle housing that dominant cows will choose to use first, such as cubicles closer to the feeding area or loafing areas with a better microclimate, and thus higher-ranking cows may have closer affiliations. However, this is not necessarily the case, because evidence suggests that social rank may not be a defining factor (Neisen et al, 2007), and research has shown that cows spent more time with those that they have formed strong social bonds with, either through being grouped together during the dry period or if they had been together from an early age (Gygax et al, 2010). Without in-depth knowledge of specific social interactions such as this, animals may be housed in groups where individuals are not compatible, which could lead to agonistic behaviours.
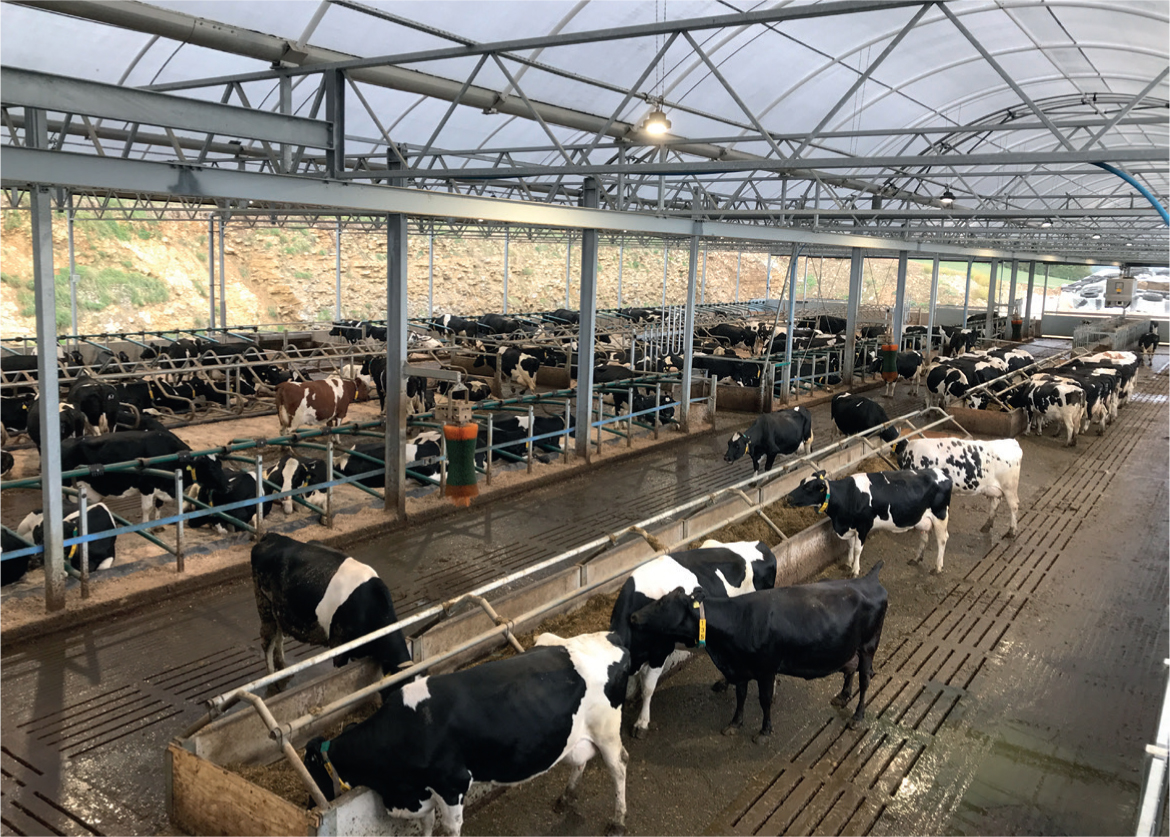
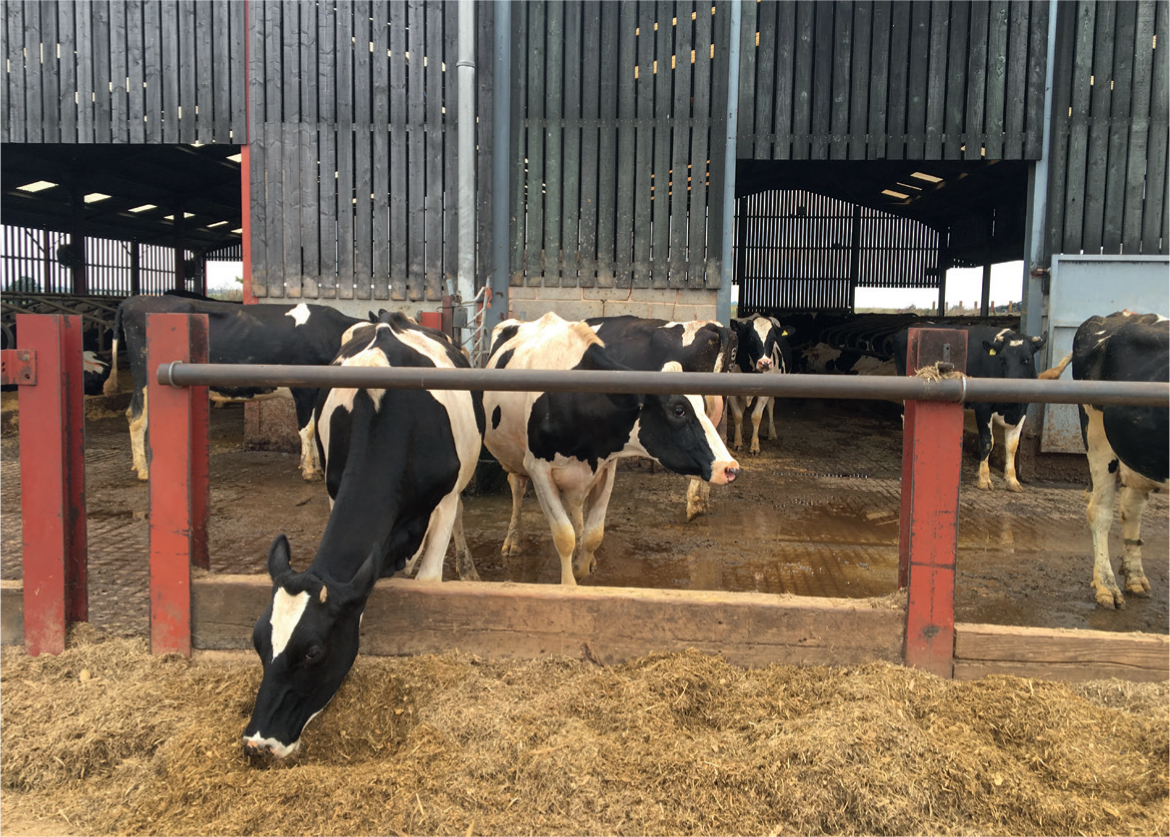
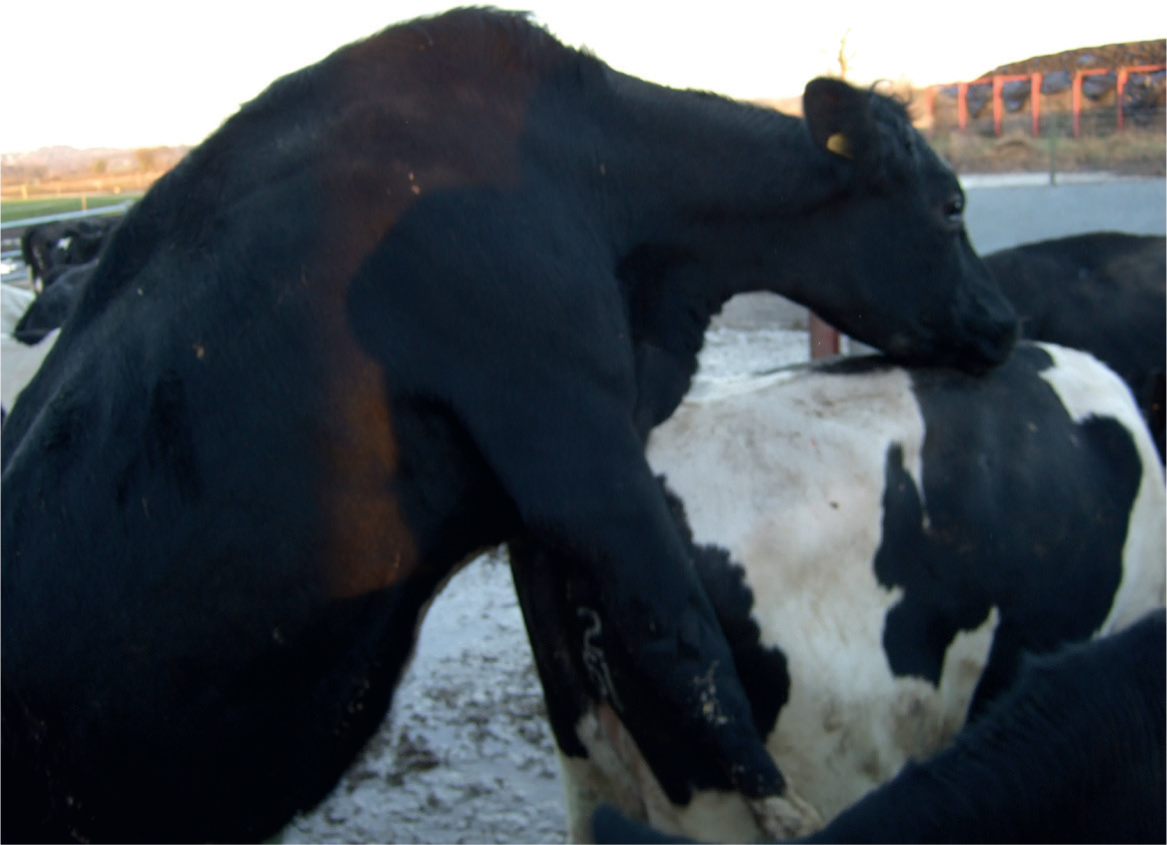
Changes in social networks are essential to consider, but it is also important to study stable networks so that farmers gain a better understanding of how animals use the facilities provided on farm. This information can then be used to make changes to housing, such as adjusting ventilation across a shed or changing feeding frequency.
How are dairy cattle typically grouped on farm?
Farm animals are kept in controlled groups, often denoted by sex, age, parity, or stage of lactation. These groupings are selected to facilitate livestock management and are therefore not natural. Dairy cow groups consist solely of females in most cases and cows within herds are likely to be a mixture of related and unrelated individuals. However, even related cows may not have interacted considerably from birth through to joining the herd, because they are usually kept separate during this 2-year period. Cattle herds are also transient, not only as a result of animals joining or leaving the herd, but also because individuals are moved to different groups depending on available infrastructure and the specific management needs of the farmer (Chebel et al, 2016). Because of the limitations associated with these groupings it is imperative that important social bonds are understood, because disrupting these bonds will lead to stress through a lack of social buffering or social support, which will have a negative impact on the welfare of cattle.
How can social network analysis be used on dairy farms?
SNA can be used effectively on farm to determine specific associations and interactions between cattle, which will help to enhance stockmanship skills. Tracking the behaviour of individuals within herds is a useful technique, but SNA can be used alongside this to show the exact associations within a herd, and the direction of behaviours toward individuals can also be assessed. The suitability of housing certain individuals together can be revealed from such analysis, and subsequent actions based on this information can reduce stress within the herd.
Proximity loggers have previously been used to analyse social networks in cattle, but there has been concerns about their accuracy (Boyland et al, 2013). However, sensor technology has become increasingly popular on farms (Neethirajan, 2017), with cows often having more than one piece of wearable technology (Figure 5), which is typically in the form of ear tags, collars or ankle monitors. Location sensor technology has developed at a fast pace and is now available on farm where cows can be tracked and easily identified, and this has an application for studying social networks of cow herds continually on commercial settings. It can provide real-time location data 24 hours a day, 7 days a week that consists of the position, movements and social interactions of individuals within dairy herds. Such technology is a relatively cost-effective method of carrying out SNA, especially if the equipment is already available on farm.
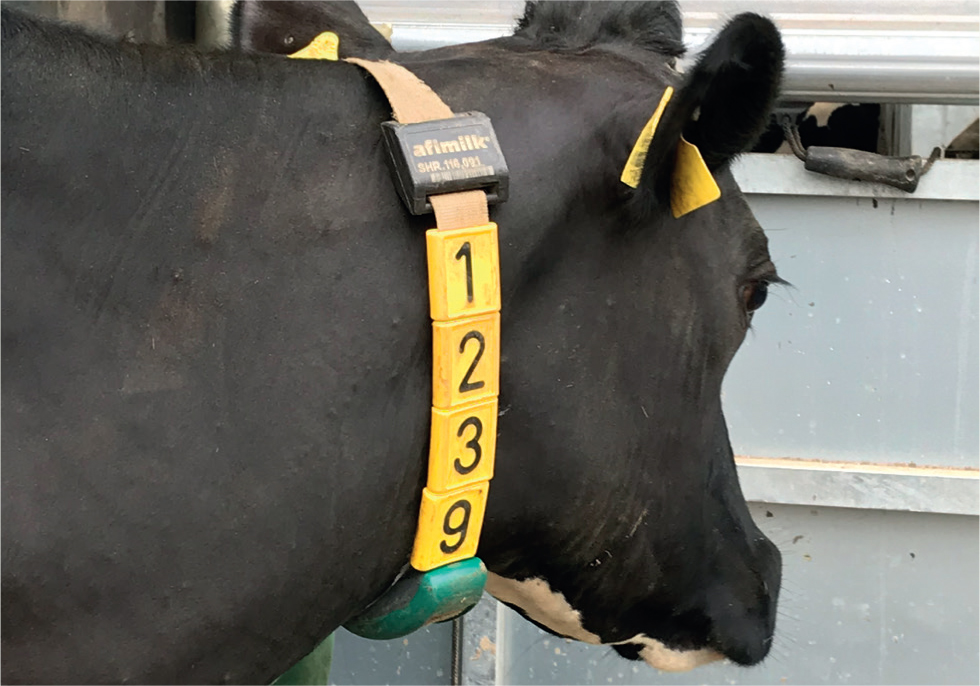
What are the benefits of using SNA on dairy farms?
SNA can be applied to understanding social interactions when stock is re-grouped. It is important that the needs of removed animals are considered, but the social structure in the remaining group will also be disrupted. Studying interactions after group changes will help determine if regrouped herds are functioning, and an understanding of associations and interactions can also be of benefit when individuals are integrated into new groups, such as the introduction of heifers into a milking group.
Some individuals within a dairy herd are likely to be key to the stability of the group and tend to be depicted in the centre of a sociogram. Determining centrality measures is an important consideration to take into account when regrouping cattle. The position of cows within a social network is also linked to their health and productivity, with evidence suggesting that more gregarious individuals have higher milk yields (Boyland, 2015).
Although the centrality of certain individuals is likely to be beneficial for group stability, those in the centre of social networks may also facilitate the spread of pathogens, which highlights the key application of the SNA approach in regard to limiting the spread of disease. Recent evidence shows that understanding cattle contact structure can reduce transmission of pathogens on farm (de Freslon et al, 2019), and SNA has been used to identify sick cows within herds. For example, research has shown that unhealthy cows have a lower level of social affiliation than healthy cows (Vimalajeewa et al, 2019), which can be identified by using this analysis. Such information can benefit farmers because it provides them with a clear indicator of illness within a herd, but there is also the potential for veterinarians to use SNA to predict the spread of disease. For instance, this technique could be used to predict the spread of mastitis within herds, or foot and mouth disease between herds. Moreover, using this approach to investigate cattle movements can provide vital information to veterinarians and help them to identify herds that are at the greatest risk of disease (Martínez-López et al, 2009; Pfeiffer et al, 2015). Farmers and veterinarians may also be able to detect oestrus more effectively by using SNA because it has been found that social affiliations increase while a cow is on heat (Vimalajeewa et al, 2019).
Conclusion
The health, welfare, and behaviour of production animals is becoming increasingly important to the consumer and therefore findings based on the SNA approach can help to provide ideal social environments for animals on farms. Understanding specific social interactions can translate into improvements of housing and enrichment on commercial enterprises, which will aid the relationship between the farming industry and consumers and promote better welfare and productivity on farm.
KEY POINTS
- Social behaviour of cattle is an important aspect of animal welfare and productivity.
- Inappropriate cattle groupings can lead to stress and agonistic behaviour.
- Social network analysis (SNA) is a tool that can be used to determine and assess specific social associations and interactions in dairy cattle herds.
- Existing technology can be used to identify and easily track interactions between individual cows.
- Evidence from SNA can highlight which individuals are central to the group, and which individuals are likely to spread pathogens.
- Understanding specific social associations and interactions can facilitate positive welfare and productivity and help to reduce the spread of disease.